How Netflix increases engagement through personalized artwork
In this edition we look how Netflix develops creative insights for promotional artwork to entice subscribers to engage with their catalog
Over the last decade, Netflix has emerged as one of the most successful companies in the entertainment industry. Founded in 1997 as a DVD rental service, Netflix has since transformed itself into a streaming giant with over 200 million subscribers worldwide. The company’s success can be attributed to its user-friendly platform, original content, and data-driven approach to content creation and recommendation. In this edition we look how Netflix develops creative insights for promotional artwork to entice subscribers to engage with their catalog.
Driving strong engagement is non-negotiable in today’s extremely competitive streaming landscape. How does Netflix create a strong set of images to pick from to create personalized artwork?
They use a top-down approach that is capable of identifying previously successful features and,
a bottom-up approach that naturally surfaces clusters of images without any preconceived notions or previous knowledge
I do a deep dive into the internal tooling Netflix built to develop data-driven recommendations and surface them to their creative strategists in an actionable, user-friendly way to create the right art for upcoming titles to boost engagement.
Why Personalize?
For years, Netflix has been working on perfecting its personalized recommendation algorithms to ensure that each of its millions of members is presented with the right titles at the right time. With a vast catalog of thousands of titles and an extensive user base of over a hundred million accounts, recommending the most suitable titles is crucial to the platform’s success. However, suggesting a title is only half the battle; it’s about getting members interested in it too. How does Netflix convince them that a title is worth watching? This is where the use of artwork and imagery comes in.
Netflix recognizes that the artwork used to represent a title is crucial to capture interest. If the image reflects something compelling, it acts as a gateway into the title and gives the viewer some visual evidence of why it might be worth watching. For example, the artwork may highlight an actor that the viewer likes, capture an exciting moment such as a car chase or contain a dramatic scene that conveys the essence of the movie. By presenting the perfect image on a member’s homepage, Netflix can increase the likelihood of them giving the title a try. Engaged users tend to translate into higher customer lifetime value (CLTV). In an environment with stiff competition, you better believe Netflix product teams will do everything they can to increase engagement!
Netflix doesn’t have one product, but rather over a hundred million different products, each tailored to an individual member with personalized recommendations and visuals.
Evolution of Netflix’s Strategy
Then: The manual scrubbing and cataloging approach
Initially, Netflix relied on a team of creative strategists and designers who developed deep knowledge about the titles to recommend and create the perfect artwork new titles. They augmented this manual approach with a set of internal tools that helped the strategists look for trends by inspecting historical artwork performance from titles that had already been launched on Netflix. However, this approach is laborious and time consuming because it quite literally involves manually scrubbing through a massive dataset of movies and shows. This approach was also prone to introducing human bias.
Now: Employing ML models
Now, Netflix leans more heavily on the data-driven approach to creating engaging promotional artwork. They do this in two ways, a top-down approach that tries to find known features that performed well historically, and a bottom-up approach that surfaces groups of images with no previous knowledge or assumptions.
Top-down approach
The top-down approach involves describing an image using attributes and identifying features that make images successful. To achieve this, they collaborate with experts who use their prior knowledge and experience to identify a wide range of features. These can include low-level features such as colour and texture, as well as higher-level features such as the number of faces, composition, and facial expressions. Pre-trained models and APIs are used to create some of these features, such as face detection and object labeling. However, for features where pre-trained models are not sufficient, Netflix builds internal datasets and models.
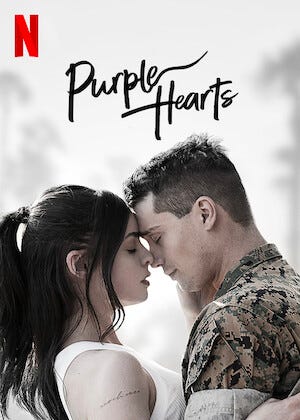
One of the challenges of creating effective artwork is determining subjective and complex attributes. To address this, Netflix has developed human-in-the-loop tools to help experts train ML models rapidly and efficiently, allowing them to build custom models for these attributes. Once an image is described using features, predictive and causal methods are used to extract insights about which features are most important for effective artwork. These insights are then used to create artwork for upcoming titles. Crazy how much work goes behind something seemingly so simple eh?
Netflix has found that certain features tend to perform better than others when it comes to artwork. For example, they discovered that single-person portraits tend to perform better than images featuring more than one person. By continuing to refine their methods and gain insights into what makes effective artwork, Netflix can better serve its members and provide them with the most personalized and engaging viewing experience possible.
For example, they discovered that single-person portraits tend to perform better than images featuring more than one person.
Bottom-up approach
The bottom-up approach does not make any assumptions or guesses about artwork. Instead, Netflix lets patterns and features bubble up naturally from the bottom and clusters images based on these patterns. You can read more about how they use neural networks to model image similarity and cluster images together in their full blog post.
Engagement Hunting?
Consider the example of how Netflix would try to personalize the artwork used to depict the movie Good Will Hunting using their top-down and bottom-up approach.
A user who has a history of watching rom-coms might be interested in watching Good Will Hunting if the artwork depicts Matt Damon and Minnie Driver. On the flip side, a user who is a fan of comedy might be drawn to watch the movie if the artwork depicted comedy royalty like Robin Williams (RIP).
For more details, read the full post on the Netflix Technology blog.